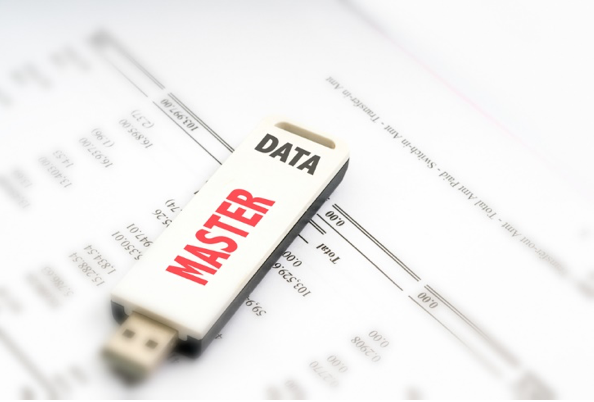
You can have data without information, but you cannot have information without data.”
– Daniel Keys Moran
The term Big Data is probably a misnomer by now, given the breadth of global data proliferation. Moore’s law used to be the oft cited benchmark around processors. That was then. While impressive, overall data growth has become the more pertinent benchmark in today’s world—perhaps Massively Big Data should be the new term.
Data growth worldwide doubles in size every two years. According to EMC, by 2020 digital data growth will reach 44 zettabytes, or 44 trillion gigabytes. Certainly impressive, and something that must keep storage engineers and data scientists up at night.
But as Daniel Moran pointed out in the quote at the beginning of this article, it boils down to this: there is a plenty of data, but very little information. Data Scientists are trying to make sense of the data sprawl, correlating data that is unstructured and disparate, with the hopes of hitting on algorithms that produce breakthroughs, or at least reveal unforeseen visual patterns that benefit both the internal enterprise and customers. Not easy, but the payoff can be substantial.
Data monetization has become one of the holy grails, and with the growth of AI, Machine Learning, and the positive disruption of transactional platforms such as blockchain, technology departments need to ensure that the data is actually clean. Without that crucial piece, the only disruption will be to the company’s reputation, and overall strategy. Trying to scrub data by virtue of gleaning the output from these tools and platforms is not the right way to cleanse the data. It is a mistake to think that “the market” will correct your data. If the issues are severe enough, trust erodes, and reputations do indeed suffer. So how can a firm move forward with these technologies, and find different ways to leverage their data assets?
Implementing a robust Master Data Management process is foundational to ensuring that firms can be agile in their approach to these forward tech adoptions, since the very core of MDM is that there is a single version of “data truth.” Organizing for MDM can admittedly be a challenge. However, in the long run, can mitigate risk, and lead to better organizational cohesiveness.
Some firms have been doing a version of MDM even before the moniker MDM came into play, since data was always at the very core of their business. Capital Markets, banking, insurance have inherent data standards for moving money and securities so they can be a bit ahead of the curve. For instance, SWIFT is one standard that comes to mind, and agreement on Securities identifier standards (CUSIP, ISIN, SEDOL, etc.) is another. But with the various data tools on the market, and unstructured and off system data now being exposed in transparent ledgers (blockchain), the MDM practice needs to evolve to become a standard in most firms. Without it, it would certainly be a challenge to leverage the firm’s data assets.
As stated earlier, MDM does take time to implement. A key part of the implementation is getting companywide buy-in and adoption, since tech does not own the data; the business lines are the data stewards as they understand how to best leverage it to solve business problems and gain opportunities. With that, the business should be involved each step of the way, since ultimately, most executives would want their company to at least be able to:
Share data and analysis intra-company, with the ability to link and add disparate and even unstructured data that is trusted.
Have a holistic view of all business entities, analyzing data as required, segmenting and combining data assets.
Provide customer intelligence internally and externally, with tools and data that allow decision support.
As a collaborative partner with the business lines, the CIO and or CDO should take the time to explain the components of an MDM implementation, especially since it can result in operational changes due to new governance policies.
So where does a firm start when there are so many moving pieces? Gartner has defined seven foundational pillars for MDM success that ensconces critical attributes:
Vision – Does the business have a defined vision? What can be done?
Strategy – Tactical or integrated?
Metrics – ROI and intra company measurements specific for each project
Governance – Shared governance based on policies, initially IT led, but needs to evolve cross enterprise. Single version of the truth!
Process – Data Life cycle, plain and simple. Mapping the full life-cycle of the data, its use cases, data rules, validations, are all required.
Technology – Start out with Extraction Transform and Load as you map out the data, and engage the business in helping to cleanse the data. Ultimately you want to arrive at the correct and agreed upon Master Data Design. Also, the selection of the right MDM tool for your enterprise is crucial.
For MDM to thrive in the enterprise, these high-level steps should be at least considered as a framework. Again, an implementation of MDM is not without its challenges, but with data expansion, and the lack of enough true information coming from a lot of data, is it prudent to ignore?
About the Author
Mark Katz has had 25+ years of experience in IT/Operations in both FinTech and InsurTech. Though he has served in several senior positions, Mark has maintained a hands-on approach to leadership. Mark has served as CIO for Financial Products and Services for XL Capital, and Global CIO for Syncora Guarantee, an IPO Carve-Out bond insurance firm. Mark holds Masters’ degrees from Columbia University and The University of Chicago. In his spare time, Mark serves on two non-profit boards, and is a professional level pianist, playing for charity and political functions.
This piece was originally published on Fintech Today: -- https://www.financialtechnologytoday.com/seven-pillars-master-data-management-success/